Towards a Skill-Based Goalie Statistic
Part Wishlist, Part Spit-balling. The Ravings of a Non-Expert
Overview of Goalie Stats
Goalies are notoriously volatile. No, not just Terry Sawchuk, Patrick Roy, or Tom Barrasso volatile. I mean inconsistent. Their results fluctuate massively based on the team in front, the opposition their facing, their own play. But we can only measure their results and hope that it’s a fair representation of their play despite all other factors.
Some stats tie up a team’s performance more than others. So right now, it’s about trying to pick the least of evils. Wins are probably the worst conventional stat to judge a goalie by since, as I’ve explained in great detail in another article, win conditions are goal-based, and goalies don’t score goals. Goals against average is also not very good. It just doesn’t tell you that much. Goals are preceded by shots, so wouldn’t it be better to measure on a shot basis? That said, it can be all you have to go by if you don’t have access to shot data. I’d call it the “bare-minimum” of all goalie stats. Save percentage is what I was previously alluding to. It’s pretty good. It tells you how many times a goalie stopped would-be goals out of all would-be goals on net. Save Percentage’s a pretty good stat, but one of its problem is that it doesn’t account for scoring environment.
In my article about Goals Save Above Average adjusted, I used this graph to critique one issue of GSAA —which we’ll get to later —which was its inability to account for season-to-season variations in average shot volume and SV%’s. It’s a little messy, but I love this graph for telling the general trend history of the NHL since 1955-56. I’d seriously consider hanging this on my wall if I could ever label it properly.
To demonstrate the era difference more tangibly, let’s look at 6 different goalie seasons:
2021-22 Robin Lehner VEG
GP: 44, .907 SV%, 2.83 GAA
2011-12 Marc-André Fleury PIT
GP 67, .913 SV%, 2.36 GAA
2001-02 Byron Dafoe BOS
GP 64, .907 SV%, 2.21 GAA
1991-92 Chris Terreri NJD
GP 54, .888 SV%, 3.18 GAA
1981-82 Greg Millen HAR
GP 55, .873 SV%, 4.30 GAA
1971-72 Roger Crozier BUF
GP 63, .903 SV%, 3.52 GAA
1963-64 Terry Sawchuk DET
GP 53, .916 SV%, 2.64 GAA
What do these seasons have in common besides being ten seasons apart (with the exception of Sawchuk)? They all have a GA%− of roughly 100, which is always league average.
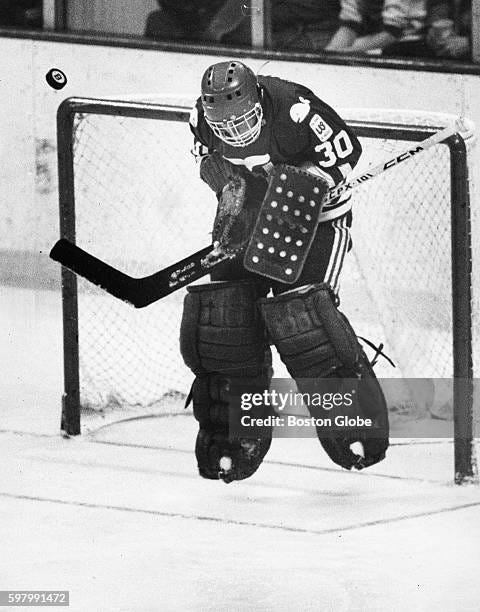
Speaking of GA%−, what the heck is that? Well, GA%− is one of my personal favourite stats. It’s basically normalized SV%. Normalized to what, you ask? Well, to 100, which as mentioned above is league average, and having a GA%− of 100−x is x% better than league average1. Except it’s not really SV%, it’s Goals Allowed %, which is 1.000−SV%. This is a minor adjustment that allows for 0 to mean “no goals allowed” which makes sense. The highest GA%− I’ve ever seen is Marc-André Fleury’s 211 (110% worse than average) from his 6 games in the 2012 playoffs. This means that the average goalie’s rate of allowing goals was closer to allowing no goals than it was to matching Marc-André Fleury’s rate.
As I mentioned before, GA%− is one of my personal favourite stats because it’s a tangible stat that’s adjusted to be comparable across eras. It gives you information about a goalie’s performance and how it compares relative to the rest of their league. But it also has its drawbacks. For example, GA%− is a rate stat and needs to be contextualized against a workload. Anthony Stolarz officially lead the NHL in GA%− in 2023-24 with 77 in 27 games played, but Frederik Andersen had a 71 GA%− in 16 games played. Neither of these should be taken fully at face value due to the smaller sample size. Sure, they did play great in the games they were in, but no ones going to argue that Connor Hellebuyck’s 80 GA%− in 60 games wasn’t deserving of the Vezina.
Now, the aforementioned GSAA is what I like to use as one of my main full-season performance indicators. It gives a clear of idea of performance within a single season by combining rate of puck-stopping with volume of shots. The formula is straightforward: (SV% x Shots against) − (League-average SV% x Shots against). A big advantage to this stat is that it has existed for as long as SV% has existed — that is, since 1955-56. But like SV% and GA%−, GSAA is blind to the quality of shots faced. This is where we can use Goals Saved Above Expected (or GSAx) to better understand what kind of shots the goalies are facing.
GSAx and Its Misaligned Expectations
While it is the best public-facing metric currently available, GSAx doesn’t directly measure goaltending. xGoals is a stat that tracks unblocked shot attempts and collects a variety of factors — shot velocity, location, time since last event (ie, unblocked shot attempt), among others — and uses them to calculate a likelihood of that unblocked shot attempt resulting in a goal. All resultant xGoals are then aggregated to represent a team’s expected offensive output.
Note that it is best to think of xGoals as a primarily offensive metric. Leon Draisaitl, for example, scores a lot of goals from bad angles. These kinds of shots typically carry fewer xGoals because they tend to miss the net more — which is one of the primary weights when calculating xGoals: if the puck is even likely to hit the net. But since Draisaitl is such a skilled shooter he can hit the net more often than xGoals would predict based on location and thus overperforms xGoals’s “xPectations”. This overperformance therefore reflects skill Draisaitl has compared to other players.
GSAx, unfortunately, doesn’t do this. GSAx’s focus is on one goalie2, yes, but let’s imagine the process of what GSAx is actually telling us on an individual play.
A shot is taken carrying a specific likelihood of success according to xGoals.
The shot is either a goal (carrying a value of 1) or not a goal (0).
“Goaltending” that is equal to the difference between the result and the expectation occurs somewhere between steps 1 and 2.
This is problematic because as we know xGoals is designed to measure offence, which requires measurement of missed nets. But that’s not relevant to goalies because missed nets aren’t shots and thus don’t require being saved. A shot on a wide-open net that misses, the same shot resulting in a desperation save, and the same desperation resulting in a missed net all result in the same GSAx.
Now, putting my goalie hat on, there is value in forcing a player to take a more difficult shot and be more likely to miss, just as there is value in deflecting passes out to the front of the net, and playing the puck.
But the problem is still that GSAx can award goalies value for shots that don’t hit the net. Fellow substacker
’s xSave model accounts for this by only using shots on goal and posts — which allows for a wider pool of data, but specifically only includes borderline misses3— as well as adding a few more factors to the mix like distance relative to the centre of the net, angle to the centre of the net as well as horizontal and vertical available angles. This is a great step forward — I’d argue it’s a better model than xGoals for goalies— but even Knodell admits that xSaves doesn’t directly measure goalie skill.So here is where I will add my thoughts to the discourse. I’m not trying to actually construct a model, per se. I don’t know how to go about that right now.
Reflecting Skill
The essence of a save is intercepting the trajectory of a puck
A Skill-Based Goalie Metric would measure a goalie’s skills and try to use those to produce a predictor of a goalie’s results. In order to understand how to measure these skills we need to ask “what do we expect good goalies to do?”
Make saves — Well, of course. The goalie’s job is to stop the puck from crossing the goal line. But what is a save? Statistically a save is any shot on goal that is not a goal. That’s not particularly useful. Shots that are going wide can still be saved. In fact, it might be more accurate to think of shots on goal as saves + goals. This prevents us from using shots on goal to define saves. So, we’ll define saves as “shot attempts4 that hit the goalie and aren’t goals”. In fact, we just need to examine own-goals to realize that goals can create shots, statistically. So let’s try a different perspective. Philosophically, the essence of a save is intercepting the trajectory of a puck. Any save is doing this in some manner. Any statistic we build to directly reflect a goalie’s skill must account for this.
Not allow a lot of goals — Kinda obvious. Or is it? Let’s look at it this way: why are all-star games typically so high-scoring? Is it because the goalies aren’t good? By definition, one would hope not. The fact that no-one really cares about defence? A bit. But how about the maximally dense concentration of the best finishers and playmakers controlling the puck at any one time? That might do it. So, if we’re trying to measure goalie skill, shouldn’t we measure goalie action and not goalie results? Well, it might be quite possible that we will inevitably tied to goalie results, but I’ll just leave it at that for now.
Not allow “easy” goals — another obvious thing when you say it out loud, but it makes sense. xGoals already has this part down: if the opponent scores on a low xGoal shot, the goalie’s xGoals suffers. But this is only as good as the model being used.
Imagine a short-side goal where the goalie is in the RVH. Some of these goals are bad goals for sure, but sometimes there’s only the slightest bit of room for the shooter to work with. So, let’s imagine two goals, both ending in a short-side, top-shelf goal, where the goalie is in the RVH. They both start off the rush, 3-on-2, puck-carrier drives wide, runs out of real estate next to the net below the faceoff circle, but decides to fire a shot high, short side and it goes in. For one goal, we’ll say the hole left open for the shooter was just over the circumference of the puck, and for the other, we’ll say the hole available was significantly larger. xGoals would say that these two goals are the same so long as they all other factors in the shot itself are equal, but the target needed for the first example is much smaller.
I’d say that the goalie in the first scenario has demonstrated more skill in executing the play — even if the result is equally negative. This is the evolutionary through-line between xGoals/xSaves to a skill-based goalie metric. xGoals predicts goals based on unblocked shot attempts. xSaves goes through similar processes, but narrows the scope to shots on goals and posts. The next step is to figure out how much effective space can a goalie cover — how good do the shots that go in have to be to go in.Be in position for shots — this is where things get interesting. Do goalies need to be in position to make saves? Trust me when I say it helps, but it’s not necessary. In fact, what we often think of as “goaltender skill” is the very ability to make these low-percentage saves. So, let’s refine this parameter to say that the best goalies are some combination of ‘in position for more saves’ or ‘able to better compensate for sub-optimal position’ than other goalies. The ‘or’ in this sentence is inclusive.
React to shots — Again, ask the same question: do goalies need to react to shots? Well, yes. But there are positions/scenarios where goalies need to react less. So, I don’t think we can necessarily isolate this factor. Besides, being in the NHL would already be something of a control for this.
Cover net — This is another important skill, but we also need to shift our perspective slightly. We can think of two types of coverages: I’ll call them ‘immediate coverage’ and ‘effective coverage’. Immediate coverage can be thought of as the area a goalie’s body occupies as well as where the butterfly would cover, whereas effective coverage can be thought of as the area where a goaltender can react to. A way of thinking about this is that immediate coverage is anywhere where allowing a goal would be described as going “through” the goalie — both literally and figuratively. Meanwhile effective coverage is basically everywhere a goalie can efficiently reach.
Let’s play with this idea a little more. We can imagine that for any angle and depth on the ice on an open net, there are a certain number of trajectories that can lead to a shot on goal. These trajectories will always form a pyramid no matter where the puck is on the ice — or in the air. So, since we know that a save is an interception of trajectory, we can imagine the immediate-coverage area intercepting a percentage of these trajectories.Made in MS Paint if it wasn’t already painfully obvious. This is actually not a new idea. I remember one goalie coach using ropes on an actual net to explain this concept to me.
Now, not all goalies have the same innate level of immediate coverage (i.e. size) but goalies can compensate for this by increasing their depth or having better “effective coverage” from the same depth. Immediate coverage is forever in trade-off with other threats. So we can assume that the best goalies will gravitate to the combination of immediate coverage and effective coverage that is generates the most success for them.Don’t give up rebounds — this one isn’t as necessary. We would assume that better goalies leave fewer rebounds. xGoals suggests that rebounds are kind of random things, so that might be the actual case. But some rebounds are more dangerous than others. If we look to the ideas of Immediate Coverage and Effective Coverage for guidance, we don’t get very clean cut answers.
Chest/body saves — the chest/torso is the platonic ideal of immediate coverage. It’s the part of the body all a goalie’s noodle-y limbs are attached to and is decidedly impermeable. What it lacks in mobility as a puck-blocking implement5 it makes up for in unmatched size. For most shots going here, there should be some expectation of entrapment because there’s little risk of pucks going through — unless the puck starts encroaching on arms territory. But again, I will admit, some parts of the chest are more difficult than others. To demarcate these areas efficiently, I will refer to the below technical diagram. Keep in mind that the orange bit is only manageable provided there is sufficient lean in the chest, directing rebounds to the ice.
Pad saves — although I’d consider pads part of immediate coverage, their mobility potential suggest otherwise. In any case, rebounds off of pads have a variety of outcomes — sometimes landing in corners, sometimes right back up the middle — but very rarely, in smothering distance. However, we can assume that there is generally a certain skill involved in directing these rebounds. Typically, you want to move from the knee and not the foot — direct, don’t kick.
Glove saves — it’s called a “trapper” for a reason (expectation of no rebounds)
Blocker saves — it’s called a “blocker” for a reason (expectation of yes rebounds).
But not all rebounds are equal. And since xGoal models insist that the capitalization of rebounds is random, goalies should be awarded/penalized based on where those rebounds land measured by relative danger based on where the puck initially hits. For example, a glove save that results in a rebound is considered less optimal than one that doesn’t. We can also have a ‘responsibility zone’ where the goalie is expected to deal with their rebounds. I’d demarcate this as an inverse ‘v-shape’ drawn from either foot in the butterfly and meeting at the point perpendicular to the midpoint between either foot, one stick-length6 from the base of the implied triangle.
Make saves in motion — This is where things get tricky. If we assume that we can get a snap shot (pun intended) of the goalie at the release of the shot and another when either a save is made or a goal is scored, we could actually create a third type of coverage — “mobile coverage” — but in order to differentiate that from effective coverage, we’d need to know if the goalie is static for the shot or in motion. But going back to the two snapshots idea, we could look at “mobile coverage” as the net gain in immediate coverage between release and save, which actually solves our distinction problem handily. This would be thrown into sharpest contrast with back-door plays requiring a lot of movement in short amount of time. But consider that for most NHL goalies — even attempting desperation saves — there is always some body part trying to intercept trajectory or force the player to release the puck on a trajectory more likely to result in a missed net by the time the puck is released. For simplicity’s sake, we might be able to categorize mobile and effective coverage together.
Coverages
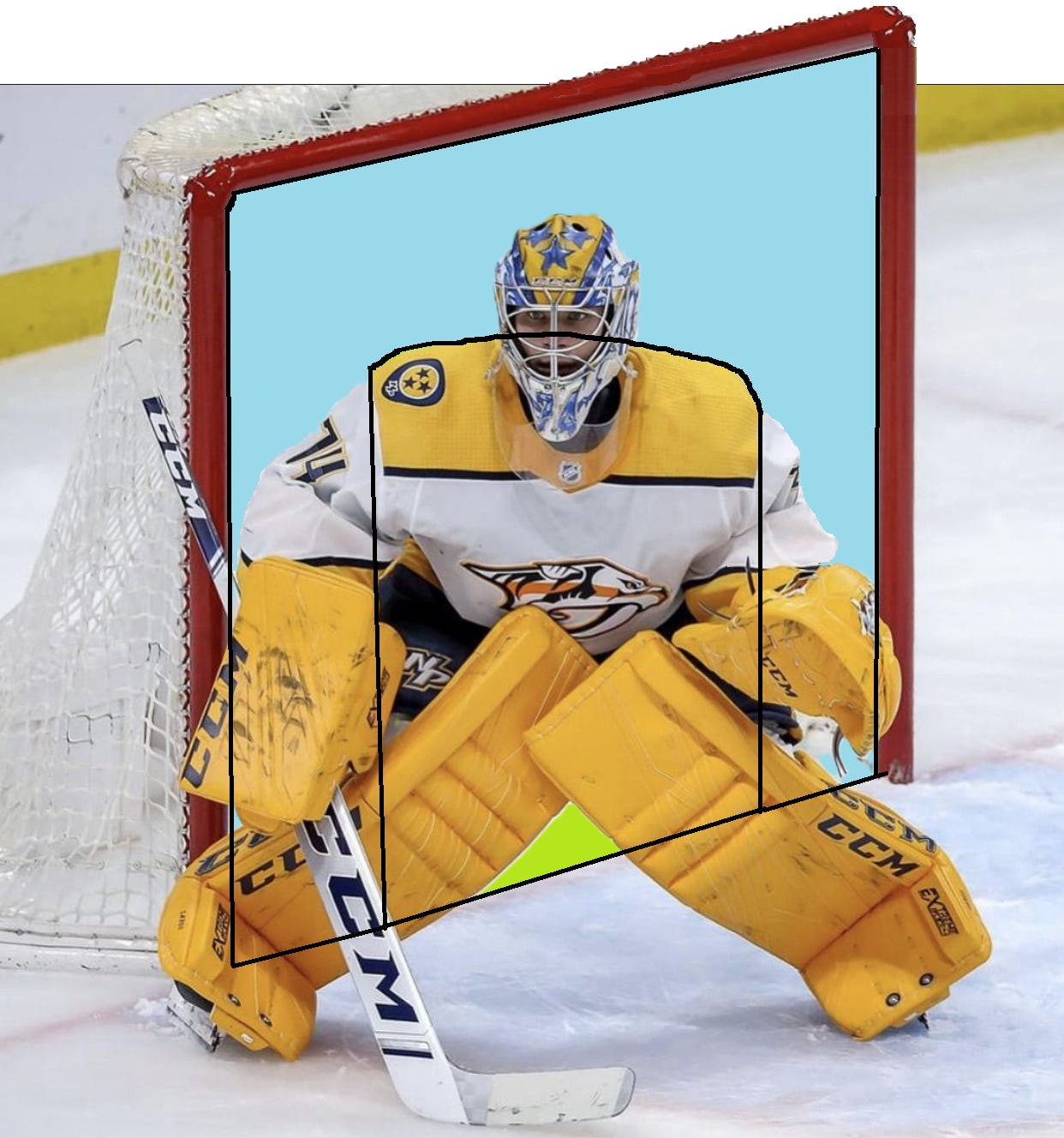
Immediate Coverage — immediate coverage is essentially all the net that a goalie covers “immediately” by their stance as well as the space that is covered by the butterfly. Getting beat by shots through the zone of immediate coverage is typically bad — albeit sometimes impossible. So perhaps there are “degrees of ‘immediacy’” ranging from the centre of the chest — which, for our purposes, we will assume is impermeable to pucks — to the 5-hole, which is not actually covered while standing or while a goalie is in movement. Immediate coverage is measured by percentage of trajectories that a goalie’s immediate coverage intercepts times whatever the “chance of permeability7” is. Making a save in the immediate coverage zone with a higher percent of coverage is worth more than an identical save with a lower percent of coverage. The difference being that the latter save is more akin to a shooter having places to score and shooting it right at the bread basket. All things equal, neither save is more difficult, but the save with higher immediate coverage will be considered better because the goalie did more before the save to increase the chance of success.
Effective Coverage — effective coverage is essentially all the net that isn’t immediately covered. However, net closer to the goalie is assumed to be more “effectively covered” than net that’s further away. Effective coverage is in direct opposition to immediate coverage
Mobile coverage — mobile coverage (not like cellular plans or whatever) is coverage gained by moving. I see two ways of treating it: (1) subcategorize it under “effective coverage” and not track it separately— which seems simpler — or (2) treat it as the retroactive difference in immediate coverage from release to moment of save. The reason this would be tracked separately is because unlike static effective coverage (e.g. a glove save where the goalie just drops into the butterfly), is the fact that goalies have a more difficult time making saves while in motion. Now, I do think we have the option to say that better goalies will be able to set their feet on more plays and/or be better at making saves in motion, thus gravitating to the combination that leads to the most success. Again, this would rely on two snapshots for every instance. I’m not to sure about the feasibility or utility of this distinction, but it might be worth making so it is worth mentioning.
Conclusion
We can think of [goalies’] actions as giving them better or worse opportunities to make saves
In writing this, it has come to my attention that teams already have models like these that they use internally. I find that both very difficult and very easy to believe. I find it easy to believe because smart teams should be trying to gain every competitive advantage possible. I find it difficult to believe because the Bruins procrastinated on signing one of the best young goalies in the game despite all signs pointing to continued success. In fact, just using the tools available to the public, I was able to determine that Swayman was more valuable last year than Charlie MacAvoy. And perhaps the Bruins knew this too, but their behaviour implies otherwise: why would you lowball one of the best goalies in the league who said he wanted to reset the market for goalies?
All that said, I don’t how much ground my ideas break, can break, or will break, but as someone who is interested in measuring goalie performance, it at the very least can’t hurt to add ideas.
This isn’t a blueprint. I think immediate coverage is the only idea that’s tangibly fleshed out: immediate coverage= % of trajectories covered by the goalie’s chest, hips, and below upon release of the puck — with the five, six, and seven hole areas being weighed against a likelihood of permeability based on distance, velocity, etc. Effective coverage, I’m a little less sure of. I guess if pressed to express it (somewhat) mathematically I’d say effective coverage is based on the likelihood of a goalie being able to cover non-immediate coverage trajectories based on factors related to the shot’s release similar (or identical) to those measured by Aaron Knodell’s xSave model.
But even if all of this is over-enthusiastic, amateurish ramblings with no basis in building a model or what current (publicly available) technologies and/or data can achieve, there are still two things to takeaway. First is my “essence of a save” observation: it might not be a radical idea, but we can internalize that any statistical analysis of goaltending action will in some way always be geometrically based. Second is the idea that we can’t only reward results, we need to also reward process. This is the only way to isolate the goalie from the opponent — if we can analyze the goalie’s actions separate from results. Goalies can’t control the shots against they face, but we can think of their actions as giving them better or worse opportunities to make saves.
Elite snipers consistently turn low-danger plays into higher-danger plays and capitalize more frequently on higher-danger plays. That is what makes them elite. Elite Goalies do the opposite: they neutralize higher-danger opportunities, but also make higher-danger chances more difficult to execute. And I’m not talking about a goalie “being in the other team’s head” because there’s no point analyzing that statistically. I’m talking about goalies more effectively covering net such that they have to beat by better — faster and more accurate— shots.
[Elite goalies] neutralize higher-danger opportunities, but also make higher-danger chances more difficult to execute
What a Skill-based Goalie Metric Would Mean
What kind of questions could we ask if we had a skill-based goalie metric? Well, perhaps we could have estimated a bit more accurately the degree to which Boston’s reliance on goaltending implied a necessity to give Swayman his bag with a bit more urgency rather than risk a season of Korpisalo as their stater. That said comparing Ottawa and Boston’s xGoals against and their GAA above expected or comparing Swayman’s Point Shares compared to his teammates both did the trick in this case.
Ideally, we would be able to better understand trends in goaltending performance — if poor results are caused by an issue in process or by the opposition being uncannily lethal.
Right now Alexandar Georgiev is generating poor results with a GSAx of -9.9 and a GSAx/60 -2.469. But last year, with a healthier sample of 3637 minutes played, he had a GSAx of 5.0 and a GSAx/60 of 0.080. So which one is real? Good question. And I’m sure the Avalanche would love to know as well. For what it’s worth, I think that Georgiev’s style isn’t very “repeatable” and his movements are very “loud” meaning he won’t always approach the same play the same way and he sometimes opens up holes when he moves (respectively). This archetype of goaltending relies a lot on timing and reaction. If those things are off, then they tend to struggle.
But until then we’re just going to have to use slightly less-educated guesses, eye-tests, and all the stats previously outlined in this article. Except for wins. Never use wins.
Reminder that x can be a negative number like −3, which would give us 103, meaning “−3% better” or, colloquially, “3% worse”
And, unavoidably, the team in front as well
Consider as well that shots that hit off the post and go in are equally counted as shots, so it accounts for that unintentional ambiguity as well.
Shot attempts being any shot released towards the goalie by an offensive player that isn’t touched by a teammate.
Welcome to goaltending: your very body is puck-blocking implement. If you can’t understand why that’s inherently fun you should probably do something else
This is just me spit-balling, but my guess for most NHL goalies is that their reach straight in front of their body while holding their stick by the paddle is about the full length of their stick from a totally upright position.
If you’re wondering why I’m using permeable, it’s because it describes something passing through an otherwise solid object. I also think it’s a funny image.
This was really, really good. You put into words a bunch of ideas that have been bouncing around in my head for a while now.
As a fellow non-expert, your breakdown of immediate, effective, and mobile coverage maps pretty neatly to my own framework for thinking about goaltending. I think goalie skill/talent can be thought of along at least four dimensions:
- Pre-shot positioning aka immediate coverage. Not just where the goalie is standing, but also arm/leg/stick positioning to most effectively cover the available net and potential deflections or redirections.
- Anticipatory positioning/positioning your body to move to cover what's left open by immediate cover aka effective coverage. How well is the goalie assessing the entire rink in front of him, the shooting danger from the puck carrier, but also the possibility of a pass and the danger from that. Do they position themselves well to cover secondary threats or do they start to cheat a bit, opening themselves up to more danger from the shooter?
- Puck tracking/reacting to how to puck moves appropriately aka mobile coverage. On a desperation save, how well does the goalie cover the area of the net the shooter is most likely to hit? On a clear shot, how well does the goalie position their pads to effectively control or redirect the rebound to a safe space?
- Reaction time. Self-explanatory, but I think slightly different from each of the positioning skills. Essentially, how quickly does the goalie move between the three and how quickly do they react when positioning is not enough to make the save.
With individual goalies being varying degrees of good at each. Obviously these aren't totally independent of each other, but I think breaking things down further increases the overlap between each dimension/skill. One skill I've left off, but that I think falls somewhere between all three types of positioning/coverage is baiting, where a goalie may appear to leave an option open to the shooter with intent of tricking them into taking that shot.
Another complicating factor is that for an individual goalie, these skills may vary by body part. Sometimes analysts talk about defensive schemes being more or less goalie-friendly, but I get the impression that these are generalities rather than defensive systems tailored to a specific goaltender's strengths and weaknesses.
And then the last complicating factor is goalie-coaching. Is the goalie being coached to play in a certain way that they have difficulty with? In theory you'd hope each goalie coach recognizes their player's strengths and weaknesses and tries to leverage the strengths to cover for the weaknesses, but I think there's no question that sometimes a goaltender is asked to play in a manner that's not intuitive to them.
I almost feel like to really evaluate goaltenders they need to be wearing like full motion capture suits with sensors at all their joints, or something like that. The next best option is probably some kind of computer vision technology that can translate multiple 2D images into a composite 3D image.
Anyway, great article! It was a fun and informative read and it sparked a lot of thoughts of my own.
I'm with Robbie here and echo his thoughts 100%. What an incredible effort on a topic that just doesn't get enough attention in my view. Not going to say I understand all of it, but immediate pure admiration for the level of effort here at bringing key insights into a tough issue to crack.
Let me offer two personal thoughts in the spirit of further discussion here. First, I've always viewed consistency of performance (I'll sometimes use the word "sustainability" as well) as indicative of a skill. On the other hand, a lack of consistency/sustainability is indicative of something that lies somewhere between pure luck and a skill. This matters in every profession - lawyers, doctors, writers, computer programmers, baristas etc. Repeatability of performance matters to something we think of as a skill.
I made this comment years ago regarding soccer goalies and their wild fluctuations in performance from year to year. That was not well received, but did not move me from my observation. Hockey goalies are similar for me. Are they skillful or somewhat lucky over the long haul? For me and other Avs fans, the question can best be restated as "was Darcy Kuemper good or lucky in 2021-2022?"
This issue ironically came back to me during the NHL Draft this year when ESPN was leaving for a commercial and the host said, "we'll be back and maybe a goalie will be drafted in the first round" - to which the voice of the analytics commentator, Meghan Chayka, in the background said "I hope not." I'm not familiar with the work of Chayka or Stathletes, but it came across as a not particularly favorable view of the skill/luck dichotomy for goalies from someone claiming to have an analytics perspective.
So, I need to be convinced at a foundational level that what goalies do rises to a real skill that can be measured and projected. That's not to say that goalies are not valuable or don't possess skillful elements in the pursuit of their performance that can matter and differentiate e.g. reflexes, positioning etc. I'm also not saying that at their higher more scrutinized level NHL goalies are not consistently better at their performance than say collegiate one or high school ones.
It's whether a valid differentiation over time exists within a level of goalies that I'm interested in understanding. Should a team pursue a supposed "great" goaltender or take a replacement level player and acquire other more impactful assets?
While some goalies can be arguably "better" than others over some period, the fluctuations in their output appear to make future projections - particularly near-term ones - very challenging. This is why it is often said that the Stanley Cup depends on having a "hot" goalie versus a highly ranked goalie during the regular season.
Finally, the economics here are perhaps a great tell. The Jeremy Swayman drama was likely not fueled by Boston being un-analytical or failing to recognize his value or his abilities or even a desire to sign him...but his relative economic value given the general variance in goaltender performance.
Swayman wanted a lot of money for a relatively thin track record of only 132 games. While I haven't examined it for some time, most goaltenders - like those in soccer - seem to not often be awarded big contracts, and some decisions to grant them are quickly regrettable. The market appears to be skittish on the position as well. In any event, the Bruins are now stuck with him - for better or worse - for 8 years at $8MM+ per.
Again, amazing and important work here that I very much hope to continue reading and enjoying. Thanks again for such a thought-provoking topic!